D-Lib Magazine
November/December 2015
Volume 21, Number 11/12
Table of Contents
Semantometrics in Coauthorship Networks: Fulltext-based Approach for Analysing Patterns of Research Collaboration
Drahomira Herrmannova
KMi, The Open University, UK
drahomira.herrmannova@open.ac.uk
Petr Knoth
Mendeley Ltd.
petr.knoth@mendeley.com
DOI: 10.1045/november2015-herrmannova
Printer-friendly Version
Abstract
To date, many studies of scientific citation, collaboration and coauthorship networks have focused on the concept of cross-community ties. In this article we explore how Semantometrics can help to characterise the types of research collaboration in scholarly publication networks and the nature of the cross-community ties, and how this information can be utilised in aiding research evaluation. In contrast to the existing research evaluation metrics such as Bibliometrics, Altmetrics or Webometrics, which are based on measuring the number of interactions in the scholarly network, Semantometrics build on the premise that fulltext is needed to understand the value of publications. Using the CORE dataset as a case study, this paper looks at the relation between the semantic distance of authors and their research endogamy value. We identify four potential types of collaboration in a coauthorship network. The results suggest similar measures can be used to provide meaningful information about the nature of collaboration in scholarly publication networks.
Keywords: Research Evaluation, Semantic Similarity, Research Endogamy, Research Publication Datasets
1 Introduction
To date, many studies of scientific citation, collaboration and coauthorship networks have focused on the concept of cross-community ties [Guimerà et al., 2005; Lambiotte and Panzarasa, 2009; Shi et al., 2010; Silva et al., 2014]. It has been observed that in citation networks bridging, or cross-community citation patterns, are characteristic of high impact papers [Shi et al., 2010]. This is likely due to the fact that such patterns have the potential of linking knowledge and people from different disciplines. The same holds true for cross-community scientific collaboration [Newman, 2004; Lambiotte and Panzarasa, 2009]. Likewise, in collaboration and coauthorship networks, it has been shown that newcomers in a group of collaborators can increase the impact of the group [Guimerà et al., 2005].
However, the studies to date have predominantly focused on analysing citation and collaboration networks without considering the content of the analysed publications. Our work focuses on analysing scholarly networks using the semantic distance of the publications in order to gain insight into the characteristics of collaboration and communication within communities.
Our hypothesis states that the information about the semantic distance of the communities will allow us to better understand the importance and the types of the cross-community ties (bridges). More specifically, in order to gain insight into the types of collaboration between authors, we are currently investigating the possibility of utilising semantic distance in a coauthorship network, together with the concept of research endogamy [Montolio et al., 2013]. In social sciences, endogamy is the practice or tendency of marrying within a social group. This concept can be transferred to research as collaboration with the same authors or collaboration among a group of authors. The concept of research endogamy has been previously used to evaluate conferences [Montolio et al., 2013; Silva et al., 2014] as well as journals and patents [Silva et al., 2014].
We refer to the set of metrics utilising fulltext for research evaluation as Semantometrics. In contrast to the traditional metrics based on measuring the number of interactions in the scholarly communication network, the premise behind Semantometrics is that fulltext is needed to understand the value of publications. In our previous work ([Knoth and Herrmannova, 2014]) we utilised this idea to develop a metric for assessing publication's contributions. Our results suggested that the semantic distance between fulltexts provides meaningful information about publication's contributions not captured by the traditional metrics. This approach is just one example of the use of Semantometrics, while the Semantometrics principles are more widely applicable.
2 Research Question
We investigate the relation that exists between the tendency to collaborate within a group of authors and the semantic distance of their respective research fields. In particular, we are interested in the distribution of the semantic distance of authors collaborating on a publication, the relation between the author distance and their endogamy value and whether, based on these two measures, there exists a typology of scientific collaboration across, and inside of, knowledge domains.
The rationale behind this approach is based on how research collaboration happens. In cases where the scientific collaboration spans fields or disciplines, that research is likely to link the two disciplines and thus provide opportunities for knowledge transfer and for novel visions and ideas [Lambiotte and Panzarasa, 2009; Silva et al., 2014]. On the other hand, collaboration within one discipline can potentially increase the authors' performance [Lambiotte and Panzarasa, 2009].
We assume that based on the combination of semantic distance and research endogamy the types of research collaboration can be divided into four groups (Table 1). We believe this classification is a useful tool in characterising the types of research collaboration that goes beyond the traditional understanding of the concept of bridges as used in scholarly communication networks. While semantic distance allows distinguishing between inter- and intra-disciplinary collaboration, research endogamy allows differentiating between emerging and established research collaborations.
Table 1: Types of research collaboration based on semantic distance of authors and their research endogamy
|
High endogamy |
Low endogamy |
High distance |
Established interdisciplinary collaboration |
Emerging interdisciplinary collaboration |
Low distance |
Expert group |
Emerging expert collaboration |
The relation between author similarity and research endogamy is studied using the CORE dataset. CORE is an aggregator of both metadata and fulltexts of open access publications from repositories and journals worldwide and covers all scientific disciplines. The availability of publication fulltexts from across disciplines enables us to perform analysis of author distance. Our results provide an overview of the publications available in the CORE dataset and an exploration of the relation between endogamy value of the publications and author distance.
2.1 Basic concepts
This section introduces basic concepts used in this article. In particular, it presents the definition of research endogamy and author distance as used in our experiment.
2.1.1 Author distance
We propose to measure the semantic distance of all coauthors of publication p as a mean value of the semantic distance of all pairs of the coauthors (Equation 1).
Here A(p) is a set of authors of publication p. Because semantic distance is a symmetric relation, this calculation can be optimised by disregarding repeating pairs in the calculation, that is by selecting the author pairs using combination rather than permutation. The number of pairs is then equal to .
We calculate the distance of a pair of coauthors by considering their publication record as a single text. The distance of two authors is then calculated by calculating the distance of two texts. While this is a very simplistic approach, it is also beneficial in terms of complexity of the calculation. Another approach would be to calculate the distance between every pair of publications of the two authors, perhaps omitting their coauthored publications. However, because the number of pair combinations of items of two sets has a polynomial growth rate, this number would significantly grow in cases of very productive authors. For this reason we chose to simplify the problem by considering publications of one author as a single text.
2.1.2 Research endogamy
In order to distinguish between emerging, short-term and established research collaboration, we propose to combine the semantic distance with the research endogamy value of the publication. The research endogamy of a publication is calculated based on research endogamy of a set of authors A, which is defined by [Montolio et al., 2013] and [Silva et al., 2014] using the Jaccard similarity coefficient (Equation 2).
Here d(A) represents papers coauthored by authors in A. A higher endogamy value then expresses more frequent collaboration. Endogamy of a publication p is then defined by [Montolio et al., 2013] and [Silva et al., 2014] as the average of endogamy values of the power set of its authors (Equation 3).
Here L(p) is the set of all subsets with at least two authors of p, L(p) = Lk(p), where Lk(p) = C(A(p), k) is the set of all subsets of A(p) of length k.
Endogamy has one significant limitation due to the way endogamy of a publication is currently defined. Because the calculation of publication endogamy is based on finding the power set of the set of publication authors, the number of times that author endogamy has be to calculated grows exponentially (this number will be exactly 2|A(p)| (|A(p)| + 1)). That means that for a publication with 20 authors, author endogamy will have to be calculated on more than 1 million sets. However, it is not uncommon to have publications with more than a thousand authors, especially in some scientific disciplines. A potential simplification could be achieved by splitting the set A(p) into groups of authors who have collaborated together on any other publication than the reference publication p, and using these subsets for the endo(p) calculation instead of using the whole set A(p). Because the reference publication p would not be considered in the calculation, this would (potentially) slightly lessen the resulting endogamy values. As the aim of this article is not redefining research endogamy, we used the existing equation, however we limited our dataset to publications with 25 or fewer authors.
3 Experiment
This section presents a basic overview of the dataset used in our experiment and the method used to obtain results. Furthermore, it provides a graphical representation of the distribution of research endogamy and author distance in the dataset and discusses the results.
3.1 Dataset
CORE (Connecting Repositories) is an aggregator of open access research papers. CORE currently aggregates both metadata and full-texts of openly accessible publications from more than 600 repositories and over 10,000 journals worldwide and from across all disciplines. For this study we have used a subset of CORE composed of:
- all full-text documents which CORE harvested from Open Research Online (ORO) repository (the Open University's repository of research publications)
- all other full-text publications found in CORE, which were authored by any of the authors of the publications harvested from ORO, added for calculating author distance and publication endogamy.
Table 2 presents overview statistics of the dataset. In the table the average number of collaborators is the mean number of different individuals an author collaborated with. The total number of publications is the number of publications in the dataset after adding all other publications found in CORE, which were authored by any of the authors from ORO. More than 4,000 publications were analysed and the whole dataset included over 30,000 publications.
Table 2: Dataset statistics.
Fulltext articles for ORO |
4,207 |
Number of authors |
8,473 |
Average number of publications per author |
7.61 |
Max number of publications per author |
310 |
Average number of authors per publication |
4.31 |
Max number of authors per publication |
25 |
Average number of received citations |
0.30 |
Average number of collaborators |
80.23 |
Total number of publications |
30,484 |
We selected the ORO repository because we needed a dataset containing the majority of publications of (at least a subset of) the academics. For this reason an institutional repository was a good candidate. We would like to note that we have not used any methods for disambiguating author names, as this problem is outside of the scope of this experiment.
3.2 Dataset processing
The following information was obtained from the CORE dataset:
- a list of authors of each of the selected documents and the publication record for each of these authors.
- the number of times the publication was cited in CORE.
- Fulltexts of the selected documents.
We calculated the author distance using the cosine similarity measure on tf-idf term-document vectors [Manning et al., 2009] created from the document full-texts. The full-texts were pre-processed by removing stop words, tokenising and stemming. The distance used in the author distance measure was then calculated as dist(d1,d2) = 1 sim(d1,d2)
, where sim(d1,d2) is the cosine similarity of texts d1 and d2 (the 1 sim(d1; d2) value is often referred to as distance although it is not a proper distance metric as it does not satisfy the triangle inequality property).
We have produced two numbers for each of the publications from the ORO repository: distance of the authors of the publication calculated according to Equation 1, and the endogamy value of the publication calculated according to Equation 3. The Python source codes used to produce this experiment are available via Bitbucket.
3.3 Results
Figure 1 presents the distribution of both calculated values: research endogamy and author distance. While the author distance is more similar to normal distribution, with mean 0.34 and standard deviation 0.19, the distribution of research endogamy is skewed with 50% of the publications having a
value of less than 0.15. This is an interesting result, as it suggests it's not that common for authors to keep collaborating with the same academics.
Figure 1: Distribution of author distance and endogamy value.
Figure 2 shows the comparison of author distance and endogamy value with the number of authors. The lines in the plot represent a linear fit of the data. There is no correlation between author distance and the number of authors (Pearson r = 0.09). There is a very slight negative correlation between endogamy value and the number of authors (Pearson r = 0.22). This is an expected behaviour because the likelihood that the endogamy value of a publication will be lower generally increases with the number of authors, however they are not directly proportional.
Figure 2: Author distance and endogamy value compared to the number of authors.
Figure 3 shows the relation between author distance and endogamy value. The lines in the plot represent the mean values of both data series. There seems to be one visible pattern in the data, which is that very few publications fall in the category of high endogamy and high author distance when using mean values as the division lines. The proportion of publications which fall into this category is 0.07, while the proportion of publications in the other categories varies between 0.27 and 0.38. This would suggest that collaboration across disciplines happens more often on a short-term basis. On the other hand, it seems that intradisciplinary research does not tend to be done in one specific way, for example researchers do not tend to collaborate more often with the same colleagues.
Figure 3: Author distance and endogamy value.
We were interested in whether certain types of publications attract more citations in general. Unfortunately the citation data was available only for a very small subset of publications. Figure 4 show the documents for which we had citation data (490 publications). The plot shows the relation between author distance and endogamy value, while the colour of the points indicates the number of received citations. The groups of publications with similar citation counts were selected based on percentile, the least cited group representing 50% of the publications while the highest cited group representing the top 10%. However, the differences between these groups are not large enough to be statistically significant. In our future work we would like to examine the relation between author distance, research endogamy and citation counts on a larger dataset.
Figure 4: Author distance, endogamy value and number of citations.
4 Conclusions
As described in this paper, we applied the Semantometric idea of using full-texts to recognise types of scholarly collaboration in research coauthorship networks. We applied semantic distance combined with research endogamy to classify research collaboration into four broad classes, and tested this classification using the CORE dataset. This classification can be useful in research evaluation studies and analytics, e.g. to identify emerging research collaborations or established expert groups. While bridges have been the concern of many research studies, their identification has been limited to structure of the interaction networks. In contrast to those approaches, our approach takes into account both the interaction network (coauthorship, citations) as well as the semantic distance between research papers or communities. This provides additional qualitative information about the collaboration, which hasn't previously been considered.
References
[1] Roger Guimerà, Brian Uzzi, Jarrett Spiro, and Luís A. Nunes Amaral. 2005. Team Assembly Mechanisms Determine Collaboration Network Structure and Team Performance. Science, 308(April):697-702. http://doi.org/10.1126/science.1106340
[2] Petr Knoth and Drahomira Herrmannova. 2014. Towards Semantometrics: A new Semantic Similarity Based Measure for Assessing a Research Publication's Contribution. D-Lib Magazine, 20(11). http://doi.org/10.1045/november2014-knoth
[3] R. Lambiotte and P. Panzarasa. 2009. Communities, knowledge creation, and information diffusion. Journal of Informetrics, 3(3):180-190. http://doi.org/10.1016/j.joi.2009.03.007
[4] Christopher D. Manning, Prabhakar Raghavan, and Hinrich Schutze. 2009. An Introduction to Information Retrieval. Number c. Cambridge University Press, online edi edition.
[5] Sergio Lopez Montolio, David Dominguez-Sal, and Josep Lluis Larriba-Pey. 2013. Research Endogamy as an Indicator of Conference Quality. SIGMOD Record, 42(2):11-16. http://doi.org/10.1145/2503792.2503795
[6] M. E. J. Newman. 2004. Coauthorship networks and patterns of scientific collaboration. Proceedings of the National Academy of Sciences of the United States of America, 101(1):5200-5205. http://doi.org/10.1073/pnas.0307545100
[7] Xiaolin Shi, Jure Leskovec, and Daniel A Mcfarland. 2010. Citing for High Impact. In Proceedings of the 10th Annual Joint Conference on Digital Libraries JCDL '10, page 49, New York, New York, USA. ACM Press. http://doi.org/10.1145/1816123.1816131
[8] Thiago H. P. Silva, Mirella M. Moro, Ana Paula C. Silva, Wagner Meira Jr., and Alberto H. F. Laender. 2014. Community-based Endogamy as an Influence Indicator. In Digital Libraries 2014 Proceedings, page 10, London, United Kingdom. http://doi.org/10.1109/JCDL.2014.6970152
About the Authors
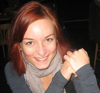 |
Drahomira Herrmannova is a Research Student at the Knowledge Media Institute, Open University, working under the supervision of Professor Zdenek Zdrahal and Dr Petr Knoth. Her research interests include bibliometrics, citation analysis, research evaluation and natural language processing. She completed her BS and MS degrees in Computer Science at Brno University of Technology, Czech Republic. Aside of her PhD she participated in research projects at the Knowledge Media Institute (CORE, OU Analyse).
|
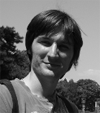 |
Petr Knoth is a Senior Data Scientist at Mendeley, where he develops text-mining tools to help researchers' workflows. Dr Knoth is also the founder of the CORE system, which aggregates millions of open access publications from repositories and journals and makes them freely available for text-mining. Previously, as a researcher at the Open University, he acted as the principle investigator on a number of national and international research projects in the areas of Text Mining and Open Science.
|
|